Abstract:
As a widely used semi-supervised learning strategy, self-training generates pseudo-labels to alleviate the labor-intensive and time-consuming annotation problems in crowd...Show MoreMetadata
Abstract:
As a widely used semi-supervised learning strategy, self-training generates pseudo-labels to alleviate the labor-intensive and time-consuming annotation problems in crowd counting while boosting the model performance with limited labeled data and massive unlabeled data. However, the noise in the pseudo-labels of the density maps greatly hinders the performance of semi-supervised crowd counting. Although auxiliary tasks, e.g., binary segmentation, are utilized to help improve the feature representation learning ability, they are isolated from the main task, i.e., density map regression and the multi-task relationships are totally ignored. To address the above issues, we develop a multi-task credible pseudo-label learning (MTCP) framework for crowd counting, consisting of three multi-task branches, i.e., density regression as the main task, and binary segmentation and confidence prediction as the auxiliary tasks. Multi-task learning is conducted on the labeled data by sharing the same feature extractor for all three tasks and taking multi-task relations into account. To reduce epistemic uncertainty, the labeled data are further expanded, by trimming the labeled data according to the predicted confidence map for low-confidence regions, which can be regarded as an effective data augmentation strategy. For unlabeled data, compared with the existing works that only use the pseudo-labels of binary segmentation, we generate credible pseudo-labels of density maps directly, which can reduce the noise in pseudo-labels and therefore decrease aleatoric uncertainty. Extensive comparisons on four crowd-counting datasets demonstrate the superiority of our proposed model over the competing methods. The code is available at: https://github.com/ljq2000/MTCP.
Published in: IEEE Transactions on Neural Networks and Learning Systems ( Volume: 35, Issue: 8, August 2024)
Funding Agency:
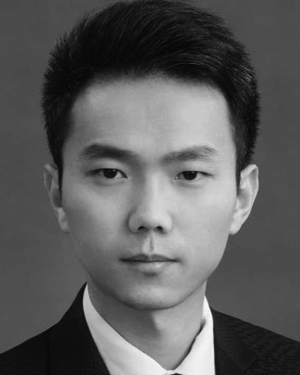
Tianjin Key Laboratory of Machine Learning, College of Intelligence and Computing, Tianjin University, Tianjin, China
Haihe Laboratory of Information Technology Application Innovation, Tianjin, China
Pengfei Zhu received the B.S. and M.S. degrees from the Harbin Institute of Technology, Harbin, China, in 2009 and 2011, respectively, and the Ph.D. degree from The Hong Kong Polytechnic University, Hong Kong, China, in 2015.
He is currently an Associate Professor with the College of Intelligence and Computing, Tianjin University, Tianjin, China. His research interests are focused on machine learning and computer vision.
Pengfei Zhu received the B.S. and M.S. degrees from the Harbin Institute of Technology, Harbin, China, in 2009 and 2011, respectively, and the Ph.D. degree from The Hong Kong Polytechnic University, Hong Kong, China, in 2015.
He is currently an Associate Professor with the College of Intelligence and Computing, Tianjin University, Tianjin, China. His research interests are focused on machine learning and computer vision.View more
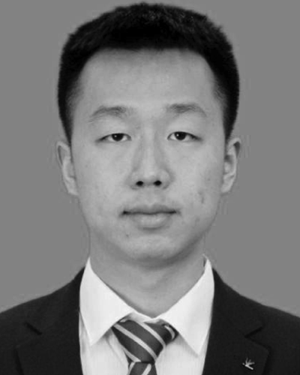
Tianjin Key Laboratory of Machine Learning, College of Intelligence and Computing, Tianjin University, Tianjin, China
Haihe Laboratory of Information Technology Application Innovation, Tianjin, China
Jingqing Li received the B.S. degree in mathematics and applied mathematics from Tianjin University, Tianjin, China, in 2021, where he is currently pursuing the master’s degree with the College of Intelligence and Computing.
His research interests include semi-supervised learning and computer vision.
Jingqing Li received the B.S. degree in mathematics and applied mathematics from Tianjin University, Tianjin, China, in 2021, where he is currently pursuing the master’s degree with the College of Intelligence and Computing.
His research interests include semi-supervised learning and computer vision.View more
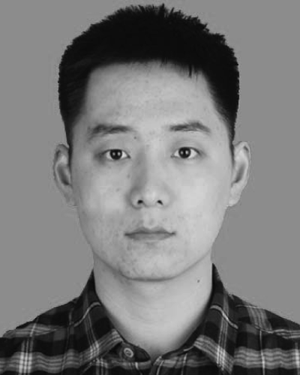
Tianjin Key Laboratory of Machine Learning, College of Intelligence and Computing, Tianjin University, Tianjin, China
Haihe Laboratory of Information Technology Application Innovation, Tianjin, China
Bing Cao received the B.S. degree in electrical engineering and automation from Hebei University, Baoding, China, in 2015, and the Ph.D. degree in information and telecommunications engineering from Xidian University, Xi’an, Shaanxi, China, in 2020.
From April 2019 to July 2020, he was a Visiting Ph.D. Student with The University of North Carolina at Chapel Hill, Chapel Hill, NC, USA. He currently works at the College of I...Show More
Bing Cao received the B.S. degree in electrical engineering and automation from Hebei University, Baoding, China, in 2015, and the Ph.D. degree in information and telecommunications engineering from Xidian University, Xi’an, Shaanxi, China, in 2020.
From April 2019 to July 2020, he was a Visiting Ph.D. Student with The University of North Carolina at Chapel Hill, Chapel Hill, NC, USA. He currently works at the College of I...View more
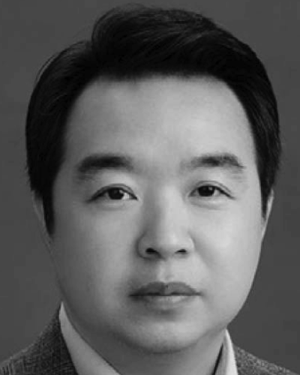
Tianjin Key Laboratory of Machine Learning, College of Intelligence and Computing, Tianjin University, Tianjin, China
Haihe Laboratory of Information Technology Application Innovation, Tianjin, China
Qinghua Hu (Senior Member, IEEE) received the B.S., M.S., and Ph.D. degrees from the Harbin Institute of Technology, Harbin, China, in 1999, 2002, and 2008, respectively.
He is currently a Professor with the College of Intelligence and Computing, Tianjin University, Tianjin, China, where he is also the Dean of the School of Artificial Intelligence. He is supported by the Key Program National Natural Science Foundation of C...Show More
Qinghua Hu (Senior Member, IEEE) received the B.S., M.S., and Ph.D. degrees from the Harbin Institute of Technology, Harbin, China, in 1999, 2002, and 2008, respectively.
He is currently a Professor with the College of Intelligence and Computing, Tianjin University, Tianjin, China, where he is also the Dean of the School of Artificial Intelligence. He is supported by the Key Program National Natural Science Foundation of C...View more
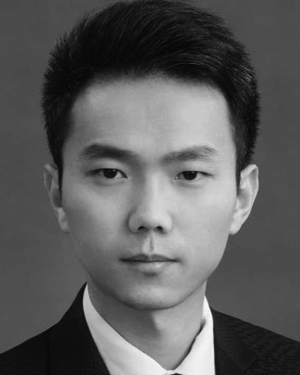
Tianjin Key Laboratory of Machine Learning, College of Intelligence and Computing, Tianjin University, Tianjin, China
Haihe Laboratory of Information Technology Application Innovation, Tianjin, China
Pengfei Zhu received the B.S. and M.S. degrees from the Harbin Institute of Technology, Harbin, China, in 2009 and 2011, respectively, and the Ph.D. degree from The Hong Kong Polytechnic University, Hong Kong, China, in 2015.
He is currently an Associate Professor with the College of Intelligence and Computing, Tianjin University, Tianjin, China. His research interests are focused on machine learning and computer vision.
Pengfei Zhu received the B.S. and M.S. degrees from the Harbin Institute of Technology, Harbin, China, in 2009 and 2011, respectively, and the Ph.D. degree from The Hong Kong Polytechnic University, Hong Kong, China, in 2015.
He is currently an Associate Professor with the College of Intelligence and Computing, Tianjin University, Tianjin, China. His research interests are focused on machine learning and computer vision.View more
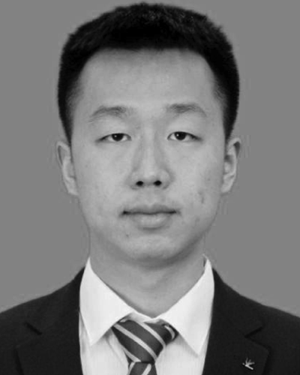
Tianjin Key Laboratory of Machine Learning, College of Intelligence and Computing, Tianjin University, Tianjin, China
Haihe Laboratory of Information Technology Application Innovation, Tianjin, China
Jingqing Li received the B.S. degree in mathematics and applied mathematics from Tianjin University, Tianjin, China, in 2021, where he is currently pursuing the master’s degree with the College of Intelligence and Computing.
His research interests include semi-supervised learning and computer vision.
Jingqing Li received the B.S. degree in mathematics and applied mathematics from Tianjin University, Tianjin, China, in 2021, where he is currently pursuing the master’s degree with the College of Intelligence and Computing.
His research interests include semi-supervised learning and computer vision.View more
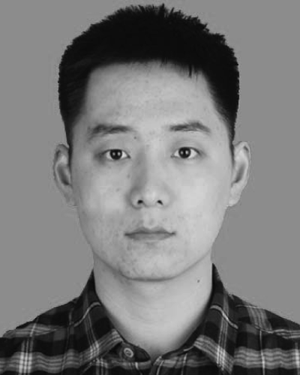
Tianjin Key Laboratory of Machine Learning, College of Intelligence and Computing, Tianjin University, Tianjin, China
Haihe Laboratory of Information Technology Application Innovation, Tianjin, China
Bing Cao received the B.S. degree in electrical engineering and automation from Hebei University, Baoding, China, in 2015, and the Ph.D. degree in information and telecommunications engineering from Xidian University, Xi’an, Shaanxi, China, in 2020.
From April 2019 to July 2020, he was a Visiting Ph.D. Student with The University of North Carolina at Chapel Hill, Chapel Hill, NC, USA. He currently works at the College of Intelligence and Computing, Tianjin University, Tianjin, China. His current research interests include computer vision, pattern recognition, and machine learning.
Bing Cao received the B.S. degree in electrical engineering and automation from Hebei University, Baoding, China, in 2015, and the Ph.D. degree in information and telecommunications engineering from Xidian University, Xi’an, Shaanxi, China, in 2020.
From April 2019 to July 2020, he was a Visiting Ph.D. Student with The University of North Carolina at Chapel Hill, Chapel Hill, NC, USA. He currently works at the College of Intelligence and Computing, Tianjin University, Tianjin, China. His current research interests include computer vision, pattern recognition, and machine learning.View more
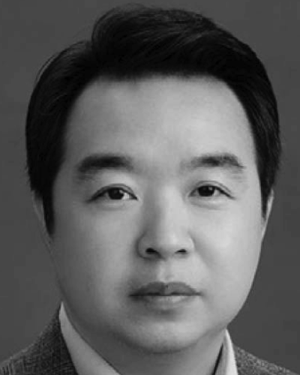
Tianjin Key Laboratory of Machine Learning, College of Intelligence and Computing, Tianjin University, Tianjin, China
Haihe Laboratory of Information Technology Application Innovation, Tianjin, China
Qinghua Hu (Senior Member, IEEE) received the B.S., M.S., and Ph.D. degrees from the Harbin Institute of Technology, Harbin, China, in 1999, 2002, and 2008, respectively.
He is currently a Professor with the College of Intelligence and Computing, Tianjin University, Tianjin, China, where he is also the Dean of the School of Artificial Intelligence. He is supported by the Key Program National Natural Science Foundation of China. He has published over 200 peer-reviewed articles. His current research is focused on uncertainty modeling in big data, machine learning with multimodality data, and intelligent unmanned systems.
Dr. Hu is the Chairperson of the Tianjin Branch of the China Computer Federation and the Vice-Director of the SIG Granular Computing and Knowledge Discovery and the Chinese Association of Artificial Intelligence. He is also an Associate Editor of the IEEE Transactions on Fuzzy Systems, Acta Automatica Sinica, and Energies.
Qinghua Hu (Senior Member, IEEE) received the B.S., M.S., and Ph.D. degrees from the Harbin Institute of Technology, Harbin, China, in 1999, 2002, and 2008, respectively.
He is currently a Professor with the College of Intelligence and Computing, Tianjin University, Tianjin, China, where he is also the Dean of the School of Artificial Intelligence. He is supported by the Key Program National Natural Science Foundation of China. He has published over 200 peer-reviewed articles. His current research is focused on uncertainty modeling in big data, machine learning with multimodality data, and intelligent unmanned systems.
Dr. Hu is the Chairperson of the Tianjin Branch of the China Computer Federation and the Vice-Director of the SIG Granular Computing and Knowledge Discovery and the Chinese Association of Artificial Intelligence. He is also an Associate Editor of the IEEE Transactions on Fuzzy Systems, Acta Automatica Sinica, and Energies.View more